As organizations strive to meet the ever-growing demand for data and analytics, they are adopting data products to streamline delivery and ensure solutions provide value to business stakeholders.
Data products are reusable data assets designed for specific uses and delivered according to agreed-upon standards and schedules. They provide tailored results and offer flexibility to cope with ever-changing data technologies and business requirements. As such, they have emerged as a key component of modern analytics strategies.
Developing data products can be challenging. The approach requires a high degree of collaboration with stakeholders. While it’s meant to streamline delivery, it also requires governance to protect quality. And there are many roles involved in creating data products that must work together.
In this article, we’ll discuss four traps that can disrupt data product development and how to avoid falling into them. These bad practices are focusing on quantity over quality, ignoring stakeholder feedback, overlooking collaboration, and putting off governance.
Four challenges of data product development
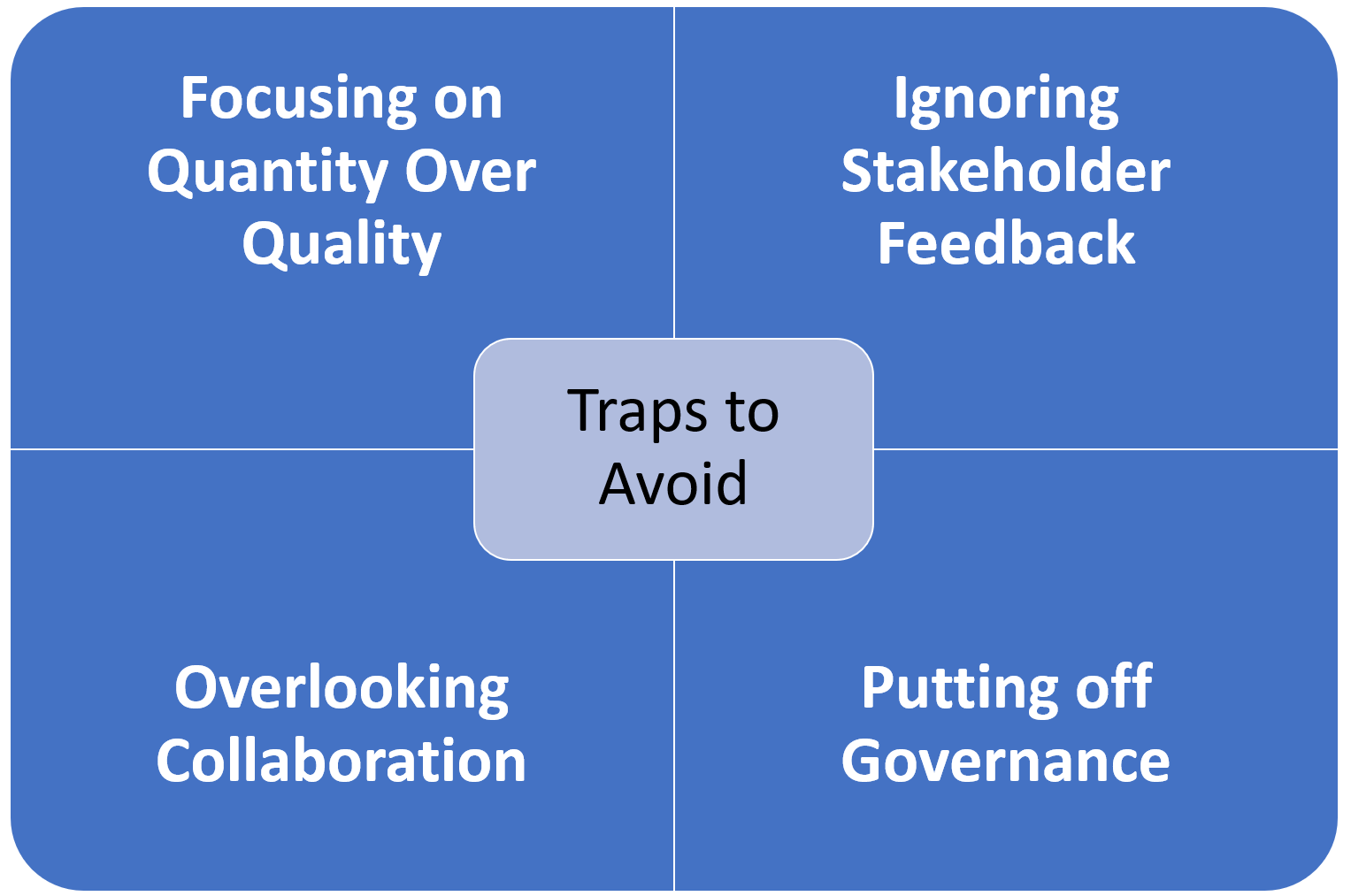
Challenge #1: focusing on the quantity of data products over quality
For most organizations, demand for data and analytics is greater than their capacity to deliver solutions. If you’re considering a data product approach with a huge backlog of requests, beware the trap of opting for quantity over quality.
The iterative approach to developing data products can make it seem as if you’re blasting through the backlog, getting lots done, and making stakeholders happy. However, that feeling of victory won’t last if your data products lack the quality and documentation to maintain your stakeholders’ trust.
Minimal Viable Data Product (MVDP). It’s important to define a set of standards that all data products must meet before a data product can be considered “done” and made available to data consumers. These common standards describe a minimal viable data product (MVDP).
MVDP standards should ensure that stakeholders can discover, understand, access, and trust the data products they use. An MVDP must include documentation about itself, such as the value it delivers, how it should be used, the product owner, the lineage of its data, service level objectives (SLOs), quality measures, sample datasets, and how to address it through an API or query interface.
Challenge #2: ignoring stakeholder feedback
To ensure you deliver a valued data product, avoid the trap of ignoring or minimizing stakeholder feedback. Any product, from a box of crayons to a luxury yacht, exists to serve a customer. When it comes to data products, data consumers are the customer.
It’s their opportunities and pain points that data products must address. You may find that the problems stakeholders initially describe are not the true issue. Their requirements will change as they understand their own needs better and as the business environment they work in changes around them.
Iterative Development. The key to managing this dynamic process is iterative development. Your stakeholders should be fully vested members of the development team, and participate in progressive rounds of product use and refinement.
As you work with them iteratively, their feedback should guide how you develop the solution. That means digging into their problems, understanding how they relate to corporate strategies and objectives, and agreeing on the expected outcomes when you solve them.
Focusing on stakeholders’ needs allows you to assess the value of your data products in terms of business outcomes.
Challenge #3: overlooking collaboration across teams
Don’t overlook collaboration across teams. To develop data, you need people with a variety of capabilities and experience including business domain knowledge, technical knowledge and skills, and product delivery skills. Since it’s unlikely that you have all those talents on one team, collaboration across teams is critical.
The product owner. A strong product owner is critical for facilitating collaboration and building a culture of teamwork necessary for successful data product delivery. The person filling this role uses a blend of strategic and tactical management to create and maintain the product vision in the form of a roadmap. The vision and roadmap helps everyone working on the product understand the value they’re helping to deliver, which is crucial for fostering teamwork.
The product owner manages the backlog of feature requests and refinements, working across teams and with stakeholders to prioritize and plan the work. This gives participants from different areas, such as data engineering, infrastructure, and information security, to weigh in on their available capacity and delivery timelines. The product owner also manages delivery by clearing roadblocks, organizing stakeholder design sessions and demos, and keeping stakeholders informed of progress.
A dedicated team. Organizations can make their data product development more effective by bringing all the players onto a dedicated data product team. This makes collaboration and teamwork easier to achieve and provides team continuity throughout the data product’s life cycle. If organizing a formal team is not feasible, a virtual team of members assigned to the product will also work.
Challenge #4: putting off governance
Earlier we discussed the trap of focusing on quantity over quality. Governance keeps you focused on quality. So don’t put off data product governance. Without it, a data product program can create a lot of siloed data with inconsistent results and documentation. It’s true that adhering to governance standards and procedures can slow down development to some extent.
However, speedy delivery is only meaningful if stakeholders can trust what’s delivered. In addition, governance helps mitigate the risk of data breaches and inappropriate use that can result in legal and financial consequences as well as costly damage to an organization’s reputation.
Automated governance. As data product programs scale, robust automated product governance becomes crucial for maintaining quality and consistency. A platform to deliver data products that automatically enforces MVDP standards can empower data product owners with self-serve publishing capabilities.
At the same time, it ensures that all products include required documentation about what it does, how it should be used, and quality measures, along with any other characteristics that governance policies specify. The platform should offer automated workflows that let users request access to a data product and explain how they intend to use it. This enables a product owner to approve or reject a request with confidence, while logging of the access requests provides details for compliance reporting.
Solutions to mitigate challenges and deliver high-quality data products
Building data products comes with challenges, as does any technology implementation. We’ve discussed four traps you can fall into and how to avoid them, summarized in the table below.
Data Product Challenges and Solutions
Trap | Solution Approach |
Focusing on quantity over Quality | Minimal viable data product (MVDP) standards |
Ignoring stakeholder feedback | Iterative development |
Overlooking collaboration across teams | A strong product owner |
Underestimating the importance of governance | Automated governance |
Using the approaches described above, you can mitigate these challenges and deliver high-quality data products. Above all remember that the point of the data product method is to deliver solutions driven by stakeholder needs. Keep that as your north star, and you’ll earn stakeholders’ trust and contribute to the overall success of your organization’s data strategy.
Eckerson Group white paper: A best practices guide to data products
Learn how data products can be implemented regardless of data architecture or methodology, giving you the flexibility to adapt to ever-changing data technologies and business requirements.