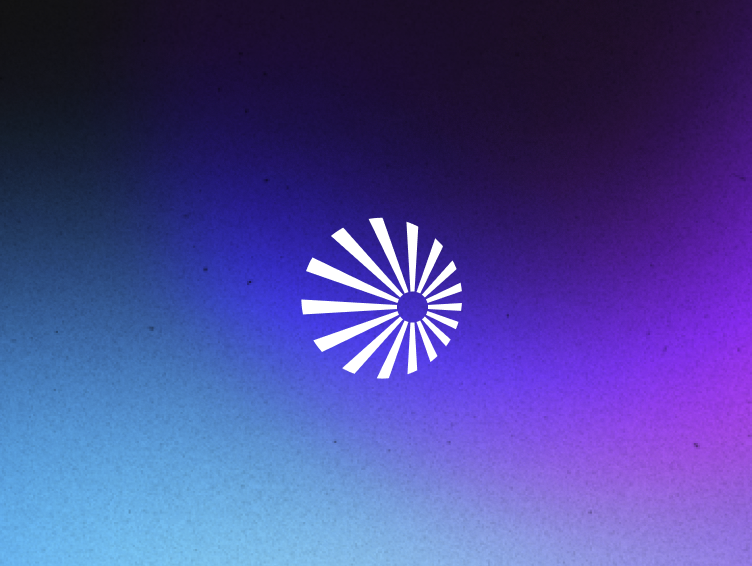
Novant Health
Case Study
Genus PLC (Genus) wanted to improve the data science lifecycle and provide instant access to more complete data. Using the Starburst query engine, the company’s data engineers were able to simplify data pipelines and directly access data across all sources for better data exploration and decision-making.
75%
faster time-to-insight
150X
faster analytical queries
150X
faster data product creation
Region
EMEA
Industry
other
Environment
aws
Solution
enterprise
Employees
1000+
Patrice Linel
Sr Manager Data Science & Data Engineering
Genus PLC
“With Starburst, we have accelerated data discovery, simplified data pipelines, and have a unified query layer across all data sources. These three points are critical to what we do.”
Genus PLC is an award-winning animal genetics company. The company researches and develops innovative animal breeding technologies that support a more sustainable food system for generations to come. Through breakthrough technologies including gene editing and reproductive biology, Genus helps farmers meet the growing global demand for food while also increasing animal well-being and sustainability in the food system.
Data engineers needed to maintain multiple databases and a hybrid data platform for genetic information and various business functions. Due to this heterogeneous environment, they were required to build and manage complex ETL pipelines that took weeks to run. Genus deployed Starburst Enterprise to improve the quality and speed of animal breeding decisions and enhance the data science lifecycle with instant access to more complete data.
Dataset interconnectivity is vital for innovation in animal breeding and genetics. Genus must maintain separate databases specialized for certain types of genetic information, such as genotypic versus phenotypic information.
The company has a data storage layer that consists of a high-performance computing (HPC) layer, a hybrid object storage layer (Azure Blob), and legacy databases for business functions. Data scientists and engineers had to query data out of multiple different systems, perform transformations on the data, and then merge and join datasets in a separate application before it could be viewed in the analytics platform. These problems resulted in slow analytical response times to ad-hoc requests, and a significant amount of work hours for engineers.
“This was a big pain for us,” explains Linel. “The main problems were associated with debugging, questionable data quality, and data provenance.”
In addition, the data science team lost an average of three days of work each time the server went down.
Linel and his team wanted to pioneer a way to solve for better, faster analytics at scale through a data mesh approach — without requiring a major shift in architecture, operations, or technology. The existing state of data management would have made analytics and machine learning at this kind of scale unachievable.
The key requirements that led Genus to select Starburst as their query engine were:
Genus chose Starburst Enterprise to support its data mesh architecture with decentralized data access and federated computational data governance. Starburst connects datasets by providing a unified query layer across all data sources. By simply implementing this tool — and without any other major system shifts — engineers can directly access data through the Starburst query engine, rather than via a complicated web of ETL pipelines.
In addition, Starburst enabled Genus to move data to less expensive platforms without disrupting data users and suspend unused clusters with autoscaling.
“When you consider all of those parameters together, that’s what Starburst gives us,” says Linel. “While other solutions, such as Databricks, were considered, none were as seamless and performant as Starburst, the fully supported, production-tested and enterprise-grade distribution of open source Trino.”
Genus deployed Starburst Enterprise and successfully accelerated its data science lifecycle while eliminating unnecessary data movement. Linel and his team experienced notable results:
Starburst also serves as the query layer across all of the company’s data sources, allowing the company to achieve faster insights into animal genetic improvement while offering a strategic solution for Genus to build its data mesh. Eventually, anyone at the company will be able to perform their own data exploration.
“Starburst plays a key role in our Data Mesh strategy,” says Linel. “It allows us to not only better integrate and adjust the governance model, but also catalog and understand data access and usage patterns.” Genus can keep its hybrid and multi-cloud data platform in sync no matter where the data pipelines reside throughout the world. “This is a huge benefit for us given that we’re a global business,” shares Linel.
This site uses cookies for performance, analytics, personalization and advertising purposes. For more information about how we use cookies please see our Cookie Policy.
These cookies are essential in order to enable you to move around the website and use its features, such as accessing secure areas of the website.
These are analytics cookies that allow us to collect information about how visitors use a website, for instance which pages visitors go to most often, and if they get error messages from web pages.
These cookies allow our website to properly function and in particular will allow you to use its more personal features.
These cookies are used by third parties to build a profile of your interests and show you relevant adverts on other sites.